An autonomous system that combines robotics with artificial intelligence (AI) to create entirely new materials has released its first trove of discoveries. The system, known as the A-Lab, devises recipes for materials, including some that might find uses in batteries or solar cells. Then, it carries out the synthesis and analyses the products — all without human intervention. Meanwhile, another AI system has predicted the existence of hundreds of thousands of stable materials, giving the A-Lab plenty of candidates to strive for in future.
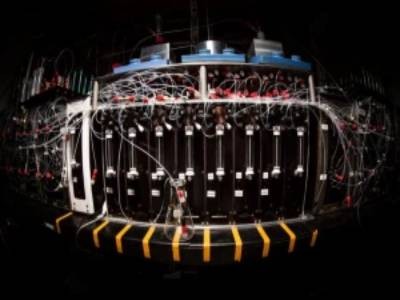
Complex molecules made to order in synthesis machine
Together, these advances promise to dramatically accelerate the discovery of materials for clean-energy technologies, next-generation electronics and a host of other applications. “A lot of the technologies around us, including batteries and solar cells, could really improve with better materials,” says Ekin Dogus Cubuk, who leads the materials discovery team at Google DeepMind in London and was involved in both studies, which were published today in Nature1,2.
“Scientific discovery is the next frontier for AI,” says Carla Gomes, co-director of the Cornell University AI for Science Institute in Ithaca, New York, who was not involved in the research. “That’s why I find this so exciting.”
Supersizing materials discovery
Over centuries of painstaking laboratory work, chemists have synthesized several hundred thousand inorganic compounds — generally speaking, materials not based on the chains of carbon atoms that are characteristic of organic chemistry. Yet studies suggest that billions of relatively simple inorganic materials are still waiting to be discovered3. So where to start looking?
Many projects have tried to cut down on time spent in the lab tinkering with various materials by computationally simulating new inorganic materials and calculating properties such as how their atoms would pack together in a crystal. These efforts — including the Materials Project based at the Lawrence Berkeley National Laboratory (LBNL) in Berkeley, California — have collectively come up with about 48,000 materials that they predict will be stable.
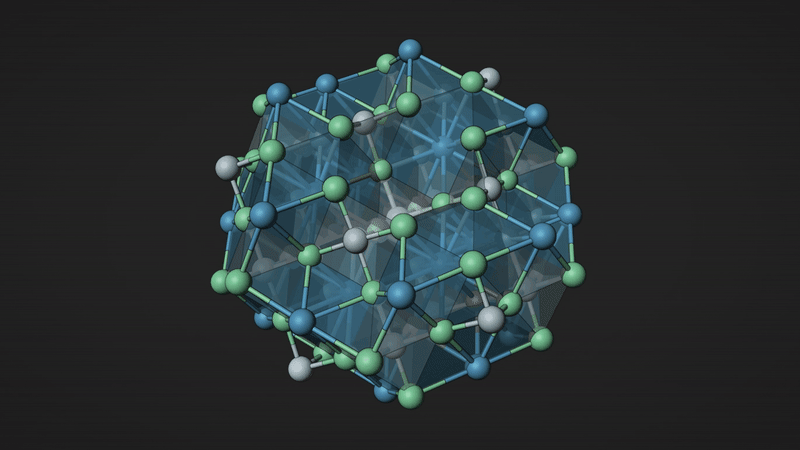
The crystal structure of Ba6Nb7O21, one of the materials predicted by GNoME. Barium is blue, niobium is grey and oxygen is green.Credit: Materials Project/Berkeley Lab
Google DeepMind has now supersized this approach with an AI system called graph networks for materials exploration (GNoME). After training on data scraped from the Materials Project and similar databases, GNoME tweaked the composition of known materials to come up with 2.2 million potential compounds. After calculating whether these materials would be stable, and predicting their crystal structures, the system produced a final tally of 381,000 new inorganic compounds to add to the Materials Project database1.
Crucially, GNoME uses several tactics to predict more materials than previous AI systems. For example, rather than changing all of the calcium ions in a material to magnesium, it might substitute only half of them, or try a wider range of unusual atom swaps. It’s no problem if these tweaks don’t work out, because the system weeds out anything that isn’t stable, and learns from its mistakes. “This is like ChatGPT for materials discovery,” Gomes says.
The indefatigable robot
It’s one thing to predict the existence of a material, but quite another to actually make it in the lab. That’s where the A-Lab comes in. “We now have the capability to rapidly make these new materials we come up with computationally,” says Gerbrand Ceder, a materials scientist at LBNL and the University of California, Berkeley, who led the A-Lab team.
The A-Lab, housed at LBNL, uses state-of-the-art robotics to mix and heat powdered solid ingredients, and then analyses the product to check whether the procedure worked. The US$2-million set-up took 18 months to build. But the biggest challenge lay in using AI to make the system truly autonomous, so that it could plan experiments, interpret data and make decisions about how to improve a synthesis. “The robots are great fun to watch, but the innovation is really under the hood,” Ceder says.
Ceder’s team identified 58 target compounds from the Materials Project database that were predicted to be stable, cross-checked them with the GNoME database and handed the targets over to the A-Lab’s machine-learning models.
By combing through more than 30,000 published synthesis procedures, the A-Lab can assess the similarity of each target to existing materials and propose ingredients and reaction temperatures needed to make it. Then the system selects the ingredients from a rack, carries out the synthesis and analyses the product. If less than half of the product is the goal material after several attempts using recipes inspired by the literature, an ‘active learning’ algorithm devises a better procedure, and the indefatigable robot starts again.
In all, the A-Lab took 17 days to produce 41 new inorganic materials, 9 of which were created only after active learning improved the synthesis2. Of the 17 materials that the A-Lab didn’t manage to make, most failed because of experimental difficulties — some materials were synthesized eventually, but only after humans intervened by, for instance, regrinding a mixture part way through a reaction.
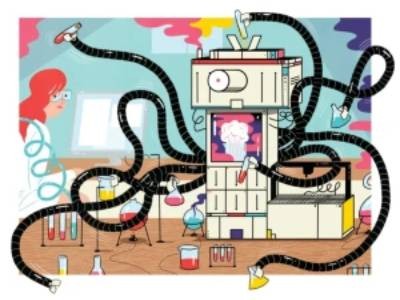
Organic synthesis: The robo-chemist
Still, it’s clear that systems such as GNoME can make many more computational predictions than even an autonomous lab can keep up with, says Andy Cooper, academic director of the Materials Innovation Factory at the University of Liverpool, UK. “What we really need is computation that tells us what to make,” Cooper says. For that, AI systems will have to accurately calculate a lot more of the predicted materials’ chemical and physical properties.
Meanwhile, the A-Lab is still running reactions and will add the results to the Materials Project, so scientists around the world can use them to inform their own work. This growing cache could be the system’s greatest legacy, Ceder says: “It’s essentially a map of the reactivity of common solids. And that’s what will change the world — not A-Lab itself, but the knowledge and information that it generates.”
Source: nature